Australia’s has the third largest marine estate of any country in the world. Much of our marine and coastal resources are in offshore or sparsely populated areas meaning that our ability to monitor and assess our environmental resources and values is particularly challenging. To maximise our understanding of our marine and coastal environment, we need to take advantage of emerging technologies and approaches.
This includes citizen science and monitoring by Indigenous communities on Land and Sea Country. In particular, it is expected that Indigenous ranger groups will utilise the same technologies and methods as mainstream science. This project has provided a series of workshops and engagement processes to best explore how to most effectively deploy technologies and community science programs to achieve maximum benefit and synergy in environmental monitoring.
Approach and findings
This project has evaluated new data collection and analysis approaches to marine monitoring of:
- pathways and barriers to emerging technology uptake by Indigenous Land and Sea managers;
- barriers to the use of drone imaging;
- citizen science as a tool for environmental management; and
- computer learning accuracy, using large citizen science, photo data sets of coral cover.
Pathways and barriers to emerging technology
Approach
By consulting with stakeholders and reviewing relevant literature we explored pathways to uptake of emerging technologies by Indigenous Land and Sea managers, by identifying: aspects of technology that support or exclude Indigenous research-leadership; and keys to broadscale adoption.
Findings and conclusions
Key principles for greater uptake, trust in, and use of emerging technologies included Indigenous- controlled: research topics, co-design, and implementation; oversight of resilient, upgradeable data systems; appropriate training; and outputs that are clear and meaningful to managers.
A pathway to achieve this must include: simplification of data collection technologies and a process for evaluating them in remote settings; development of local skills and expertise; and, a process for clarifying the real cost of building managing and improving technology solutions. Technologies that support ecosystem-service accounting are also important to support carbon and environmental market participation.
Drone imaging
Approach
Drone-supported imaging is already used in many locations and has great potential to cost-effectively address monitoring and mapping requirements elsewhere on Country. To expand uptake, a better understanding of barriers to drone use was obtained through a systematic review of relevant literature.
Findings and conclusions
A suite of barriers was identified, fitting nine categories: limitations in technology; analytical and processing; regulatory; cost; safety; social; wildlife impacts; suitability for the task; and, ‘other’.
The findings set the foundation for further evaluations of issues associated with drone use for research and management purposes, and provide a first step toward the development of potential mitigation strategies.
Citizen science
Approach
Citizen science is data collected by non-expert volunteers as written or photographic records, for a data-base designed by an overseeing organisation. The large potential pool of community researchers, and relatively low cost, make citizen science a promising and attractive proposition for the collection of environmental information, particularly over large study areas.
Opportunities and limitations of citizen science were assessed by consulting with marine managers, scientists and others experienced in collecting, collating and utilising this type of data.
Findings and conclusions
Experts agreed that citizen science data is still an emerging research field, and there are problems to resolve before it can be confidently used by managers and other end-users. Inherent biases exist within the data that are largely unknowable because of the diverse condition under which the data is collected. It is potentially very useful, however, for filling gaps within systematic surveys, and in strengthening population trend analyses, at relatively low cost.
Machine learning
Approach
Great Reef Census’ photographic records of coral cover and reef structure, were collected by volunteers, and then utilised to test the accuracy of interpretative machine learning (Artificial Intelligence; AI) in analysing coral cover and coral type in images.
Findings and conclusions
The developed machine learning platform has good levels of interpretative accuracy in identifying coral cover and coral type in images. In most cases, it has a high accuracy of ± 5 % when using just 20 images. Lesser accuracy occurs when coral cover is relatively high or low, but this can likely be rectified by obtaining more images at a particular location. Despite this, AI image analysis has great potential for producing reliable interpretative outputs from the vast census data set.
Outcomes
- Better understanding of research methods to support planning.
- Evaluation of technological innovations, and recommendations.
- Indigenous-led research supported.
Project location
Northern Australia Kimberleys to Cape York
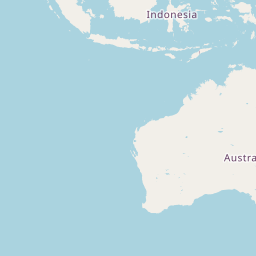

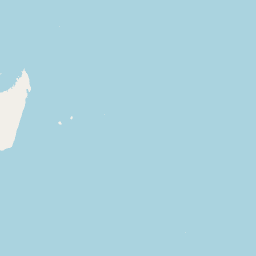

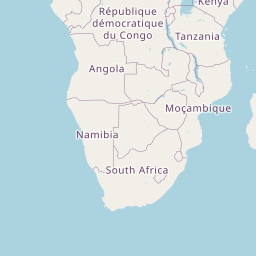
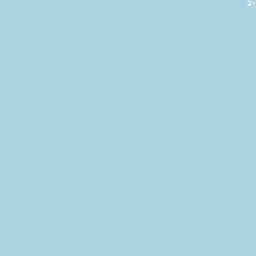
Project leader
Research partners
James Cook University
Research users
Land and sea rangers
Traditional Owners
Research community
Non-profit organisations
Department of Climate Change, Energy, the Environment and Water
Technical report – Project 1.29
Great Reef Census – a case study to integrate citizen science data into research output for marine habitat management
Lawson C. L., Mumby P., Roelfsema C., Chartrand K., Kolluru A., Shi H., Tan A., Yuan Toh Z., Ganesan C., Navindra Kothalawal V., Iyengar A., Senn N., Vozzo B., Uusitalo J. and A. Ridley, 2023. Great Reef Census - a case study to integrate citizen science data into research output for marine habitat management. Report to the Reef and Rainforest Research Centre, Cairns, Queensland.
Technical report – Project 1.29
Defining a pathway for the operational use of emerging technologies on Country
Perry J, Perdevich J, McCreedy E, and Pearse M (2023). Defining a pathway for the operational use of emerging technologies on Country. Report to the National Environmental Science Program. NAILSMA. Cairns, Queensland.
Journal article – Project 1.29
Barriers to using UAVs in conservation and environmental management: a systematic review
Walker SE, Sheaves M, and Waltham NJ (2022). Barriers to using UAVs in conservation and environmental management: A systematic review. Environmental Management 71, 1052–1064. https://doi.org/10.1007/s00267-022-01768-8
Technical report – Project 1.29
New approaches to monitoring: maximising the utility of opportunistic and citizen science datasets for conservation management
Evans K, Patterson T, Peel D (2023). Scoping study: New approaches to monitoring. Subcomponent: Maximising the utility of opportunistic and citizen science datasets for conservation management. Report to the National Environmental Science Program. CSIRO.